AI Risks: Exploring the Critical Challenges of Artificial Intelligence
Understand the potential benefits and critical risks of artificial intelligence (AI).
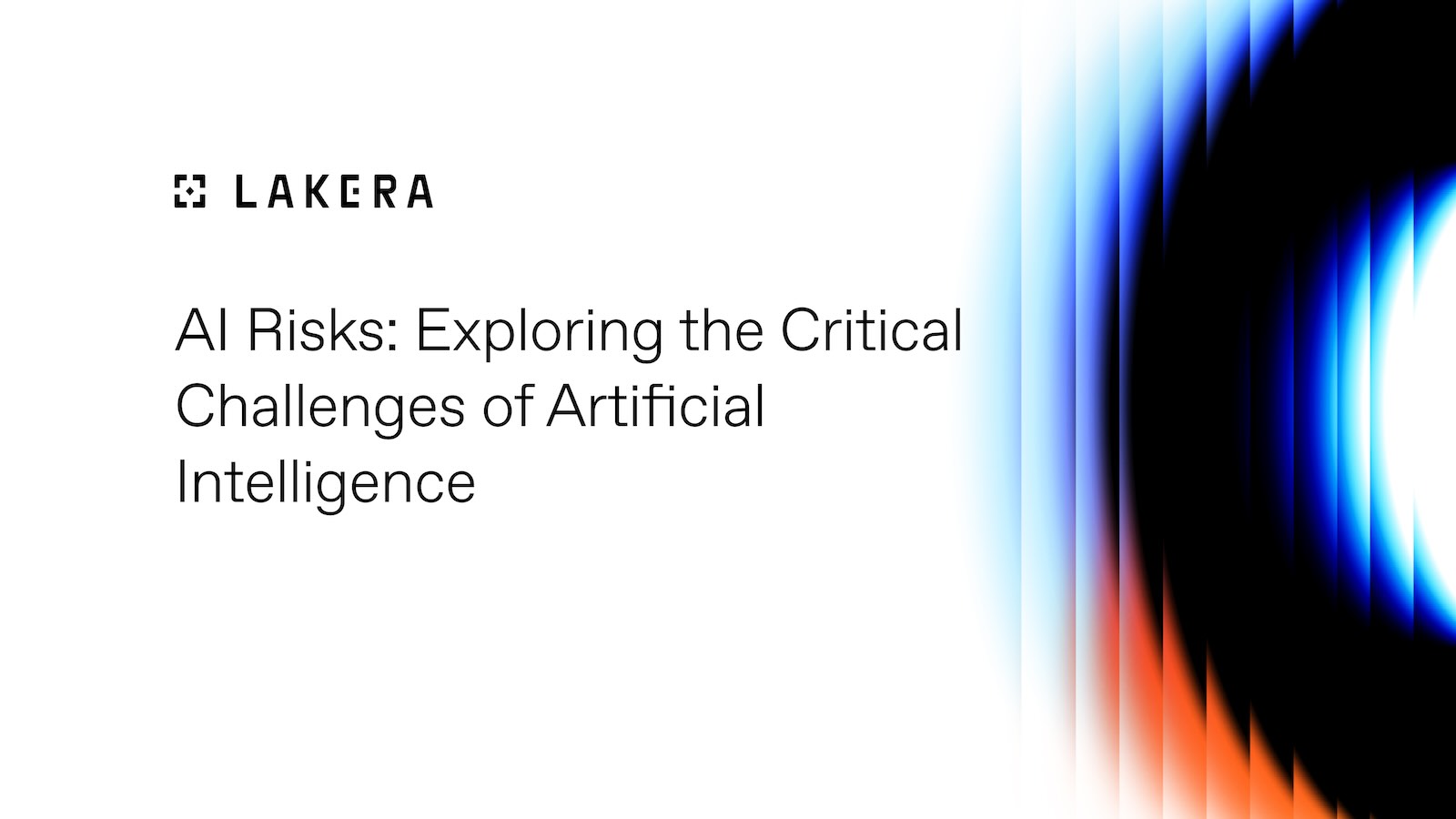
Understand the potential benefits and critical risks of artificial intelligence (AI).
Download this guide to delve into the most common LLM security risks and ways to mitigate them.
In-context learning
As users increasingly rely on Large Language Models (LLMs) to accomplish their daily tasks, their concerns about the potential leakage of private data by these models have surged.
[Provide the input text here]
[Provide the input text here]
Lorem ipsum dolor sit amet, consectetur adipiscing elit. Suspendisse varius enim in eros elementum tristique. Duis cursus, mi quis viverra ornare, eros dolor interdum nulla, ut commodo diam libero vitae erat. Aenean faucibus nibh et justo cursus id rutrum lorem imperdiet. Nunc ut sem vitae risus tristique posuere.
Lorem ipsum dolor sit amet, Q: I had 10 cookies. I ate 2 of them, and then I gave 5 of them to my friend. My grandma gave me another 2boxes of cookies, with 2 cookies inside each box. How many cookies do I have now?
Title italic
A: At the beginning there was 10 cookies, then 2 of them were eaten, so 8 cookies were left. Then 5 cookieswere given toa friend, so 3 cookies were left. 3 cookies + 2 boxes of 2 cookies (4 cookies) = 7 cookies. Youhave 7 cookies.
English to French Translation:
Q: A bartender had 20 pints. One customer has broken one pint, another has broken 5 pints. A bartender boughtthree boxes, 4 pints in each. How many pints does bartender have now?
Lorem ipsum dolor sit amet, line first
line second
line third
Lorem ipsum dolor sit amet, Q: I had 10 cookies. I ate 2 of them, and then I gave 5 of them to my friend. My grandma gave me another 2boxes of cookies, with 2 cookies inside each box. How many cookies do I have now?
Title italic Title italicTitle italicTitle italicTitle italicTitle italicTitle italic
A: At the beginning there was 10 cookies, then 2 of them were eaten, so 8 cookies were left. Then 5 cookieswere given toa friend, so 3 cookies were left. 3 cookies + 2 boxes of 2 cookies (4 cookies) = 7 cookies. Youhave 7 cookies.
English to French Translation:
Q: A bartender had 20 pints. One customer has broken one pint, another has broken 5 pints. A bartender boughtthree boxes, 4 pints in each. How many pints does bartender have now?
Artificial intelligence (AI) has swiftly transitioned from a futuristic concept to an integral part of everyday life. From virtual assistants to recommendation algorithms, AI has become the new normal, permeating industries and revolutionizing how we interact with technology. While the transformative potential of AI is undeniable, so too are the multifaceted risks it presents.
As we harness the power of AI to automate tasks, optimize processes, and even make decisions, we simultaneously expose ourselves to a host of inherent risks like algorithmic bias or the potential for AI-driven misinformation or job displacement. The growing importance of AI risk management cannot be overstated. As AI becomes increasingly intertwined with our daily lives and critical systems, it becomes imperative to understand, anticipate, and mitigate the risks associated with its deployment.
In this article, we delve into the multifaceted risks of artificial intelligence, exploring its transformative potential while shedding light on the crucial need for robust AI risk management strategies in our increasingly AI-driven world.
{{Advert}}
Here we take a look into a structured exploration of AI risks, starting with broad categories encompassing technical, ethical, and societal dimensions. It then delves into specific AI risks across different domains and applications. Lastly, it focuses on the unique vulnerabilities posed by LLMs. By categorizing AI risks in this manner, stakeholders can better understand the diverse challenges and develop targeted strategies to address them effectively.
The impact on labor income will predominantly be determined by the degree to which AI complements high-income workers. Should AI significantly enhance the productivity of higher-earning individuals, it may result in a disproportionate increase in their labor income. Furthermore, the productivity gains realized by firms adopting AI are likely to bolster returns on capital, which may further benefit high earners. These dual phenomena have the potential to exacerbate socioeconomic inequality.
The risks posed by LLMs extend beyond individual applications to encompass broader organizational and societal implications. Improper usage of LLMs, like ChatGPT, can lead to organizational vulnerabilities, such as inadvertent disclosure of sensitive information during conversations.
With LLMs deeply integrated into various sectors and daily interactions, their associated risks are widespread. As one of the most pervasive forms of AI in society today, discussions about AI risks are often synonymous with discussions about LLM risks. Addressing LLM risks comprehensively is essential for mitigating broader organizational and societal risks stemming from their widespread adoption and utilization.
The Open Web Application Security Project (OWASP) has compiled a list of the top 10 vulnerabilities commonly found in LLM applications. This compilation highlights their significant impact, vulnerability to exploitation, and widespread occurrence in real-world applications. The purpose of this list is to increase awareness of these vulnerabilities, suggest practical remediation approaches, and ultimately improve the security of LLM applications.
**💡Pro Tip: Learn how Lakera’s solutions align with OWASP Top 10 for LLMs.**
The rapid advancement of Artificial Intelligence (AI) raises significant ethical and societal concerns. These include issues of privacy, bias, transparency, job displacement, algorithmic discrimination, and autonomous decision-making. Addressing these challenges requires robust ethical frameworks, regulatory oversight, and public engagement to guide responsible AI development and deployment. Prioritizing values such as fairness, accountability, transparency, and human dignity is essential to ensure that AI benefits society while minimizing harm.
When we speak of ethical issues of AI, there tends to be an implicit assumption that we are speaking of morally bad things. And, of course, most of the AI debate revolves around such morally problematic outcomes that need to be addressed. However, it is worth highlighting that AI promises numerous benefits. Many AI policy documents focus on the economic benefits of AI that are expected to arise from higher levels of efficiency and productivity.
The International Risk Governance Center (2018) commends AI’s analytical prowess, i.e. the ability to analyze quantities and sources of data that humans simply cannot process. AI excels in linking data, identifying patterns, and generating insights across diverse domains and geographic boundaries. Its consistency and adaptability enable it to swiftly respond to changing inputs, thereby liberating humans from tedious or repetitive tasks. These technical capabilities are inherently conducive to human flourishing as they foster a deeper understanding and provide invaluable insights into various phenomena.
For example, Jeff Schneider, the Engineering Lead for Uber ATC, highlighted in an NPR interview the company's utilization of machine learning to forecast rider demand, aiming to eliminate the need for "surge pricing." Surge pricing entails short periods of sharp price hikes to reduce rider demand and boost driver availability. Uber's Head of Machine Learning corroborated the use of ML in various aspects such as estimating ride arrival times (ETAs), predicting meal delivery times on UberEATS, determining optimal pickup locations, and detecting fraud through heat maps.
But ethical risks of AI models are also no joke. A primary and frequently cited ethical issue is that of privacy and data protection. AI based on machine learning poses several risks to data protection. On the one hand it needs large data sets for training purposes, and the access to those data sets can raise questions of data protection. More interesting, and more specific to AI, is the problem that AI and its ability to detect patterns may pose privacy risks, even where no direct access to personal data is possible.
While reliability is a concern for all technical artifacts, the opacity of machine learning systems and their unpredictability mean that traditional deterministic testing regimes may not be applicable to them. The outputs of machine learning systems depend on the quality of the training data, which may be difficult to ascertain.
For example, an AI system used for the identification of disease markers in pathology may work well under research conditions, with well-labeled training data, and perform at the level of a trained pathologist, or even better, under such conditions. This does not guarantee that the same system using the same model would perform as well under clinical conditions, which may be one of the reasons why, despite the great promise that AI holds for medicine, there are relatively few AI systems already in clinical practice according to this 2019 study.
Responsible AI practices are thus essential for AI deployment. Google AI outlines a set of guidelines that should be followed for such responsible AI deployment. OWASP has also curated a list of the 10 most critical vulnerabilities frequently observed in Large Language Model (LLM) applications. Additionally, MITRE ATLAS (Adversarial Threat Landscape for AI Systems) serves as a comprehensive and globally accessible repository, providing real-world insights into adversary tactics and techniques observed through actual attacks and realistic demonstrations by AI red teams and security groups.
**💡Pro Tip: Learn how Lakera proactively mitigates significant risks associated with adversarial AI, as identified by the ATLAS framework.**
In healthcare, AI technologies offer unprecedented opportunities for diagnosis, treatment optimization, and personalized medicine. From medical imaging analysis to drug discovery, AI has the potential to revolutionize patient care, improving outcomes and reducing costs. Similarly, in the banking sector, AI facilitates efficient fraud detection, risk assessment, and personalized financial services.
Chief Risk Officers (CROs) at global systemically important banks (G-SIBs) were more likely to focus on automation (67%) and financial crime monitoring (50%) in their AI/ML deployments than non-G-SIBs. Over time, the adoption of AI-enabled scenario modeling is expected to expand, encompassing market simulation, portfolio optimization, and credit risk assessments within the banking sector. Another avenue through which banking CROs are likely to embrace generative AI is the automation of model documentation, aimed at ensuring consistency, clarity, and reproducibility across their operations.
However, alongside these benefits, understanding the risks associated with AI is imperative for fostering trust and acceptance within society. AI systems are not immune to biases, errors, or unintended consequences, which can have profound implications, particularly in critical sectors like healthcare and finance.
A recent example is IBM Watson Health’s cancer AI algorithm (known as Watson for Oncology). Used by hundreds of hospitals around the world for recommending treatments for patients with cancer, the algorithm was based on a small number of synthetic, non-real cases with very limited input (real data) of oncologists.
Many of the actual output recommendations for treatment were shown to be erroneous, such as suggesting the use of Bevacizumab in a patient with severe bleeding, which represents an explicit contraindication and ‘black box’ warning for the drug. This example also highlights the potential for major harm to patients, and thus for medical malpractice, by a flawed algorithm. Instead of a single doctor’s mistake hurting a patient, the potential for a machine algorithm inducing iatrogenic risk is vast. This is all the more reason that systematic debugging, audit, extensive simulation, and validation, along with prospective scrutiny, are required when an AI algorithm is deployed.
Thus, all AI frameworks need to be continuously reviewed and evolved to support generative AI and address the incremental risks associated with this technology—including hallucinations (where the models create fictitious responses) and bias management.
As AI continues to advance at a rapid pace, its integration into various aspects of society brings forth a multitude of potential risks and challenges. In this section, we delve into some of the most pressing AI risks, examining their implications and exploring strategies for mitigation.
One of the most pressing issues surrounding AI revolves around the possibility of extensive job displacement and automation. AI systems demonstrate exceptional proficiency in executing routine and repetitive tasks, often surpassing human capabilities, thereby paving the way for the automation of roles across diverse sectors. Occupations involving tasks such as data entry, customer service, and basic analysis are especially susceptible to this transformation. For instance, the deployment of chatbots and virtual assistants has streamlined customer inquiries and support, diminishing the necessity for human intervention. Goldman Sachs recently predicted that companies would use it to eliminate a quarter of all current work tasks in the United States and Europe, meaning tens of millions of people could lose jobs.
According to Harvard Business Review, there are two ways to deal with this risk. One option is for governments to step in, although it's unlikely they'd slow down AI adoption. Instead, they might provide special welfare programs to help and retrain those who lose their jobs due to automation.
Another approach, often overlooked, involves companies. Some are quickly integrating generative AI into their systems not just to automate tasks, but to empower employees to do more. This could lead to a big change in how companies operate, sparking new ways to create value. If many companies go this route, we might generate enough new jobs to avoid the short-term displacement problem.
AI systems are also not immune to biases that crawl in from the data they were trained on. This has significant implications for hiring practices, where AI-powered resume screening algorithms may inadvertently discriminate against certain groups. Ensuring ethical and unbiased AI systems requires careful testing, evaluation, and ongoing monitoring.
Social manipulation and surveillance refer to the use of AI technologies to influence and monitor individuals' behavior, opinions, and interactions within society. This encompasses various tactics, such as targeted propaganda, psychological manipulation, and intrusive monitoring of online activities.
This is a significant risk due to the potential for AI-powered algorithms to exploit vulnerabilities in human psychology and manipulate individuals' perceptions and decision-making processes. Moreover, the widespread deployment of surveillance technologies powered by AI raises concerns about privacy infringement and the erosion of civil liberties.
The implications of social manipulation and surveillance are profound, encompassing threats to democracy, individual autonomy, and societal cohesion. By exploiting AI's ability to analyze vast amounts of data and identify patterns, malicious actors can disseminate misinformation, sow discord, and undermine public trust in institutions. Furthermore, the proliferation of surveillance technologies equipped with AI algorithms poses risks of mass surveillance, enabling governments and corporations to monitor and control individuals' behavior with unprecedented precision.
To mitigate the risks associated with social manipulation and surveillance, comprehensive regulatory frameworks and ethical guidelines must be established to govern the use of AI technologies. This includes transparency requirements for AI algorithms, robust data privacy protections, and mechanisms to hold accountable those responsible for malicious manipulation or surveillance activities.
During the 2016 and 2020 presidential elections, AI-powered algorithms were used to amplify disinformation campaigns (for example creating deceptive videos of Biden or Trump sharing deepfake videos), manipulate public opinion, and polarize society. Additionally, the widespread adoption of AI-driven surveillance technologies, such as facial recognition systems and predictive policing algorithms, has raised concerns about privacy violations and discriminatory practices. For instance, the use of facial recognition technology by law enforcement agencies has been criticized for its potential to disproportionately target marginalized communities and perpetuate racial biases.
These examples underscore the urgent need for proactive measures to address the risks posed by social manipulation and surveillance enabled by AI technologies.
Data Privacy and AI Bias encompasses the intersection of two critical issues: the protection of personal data and the potential biases inherent in artificial intelligence (AI) systems. The risk arises from several factors. Firstly, AI algorithms rely heavily on vast amounts of data to learn and make decisions. If this data contains biases or reflects historical inequalities, the AI model may inadvertently learn and perpetuate these biases. Additionally, the opaque nature of many AI algorithms makes it challenging to identify and mitigate bias effectively.
Biased AI systems can lead to discriminatory outcomes in domains like employment, finance, healthcare, and criminal justice. For example, biased AI algorithms used in hiring processes may disproportionately disadvantage certain demographic groups.
A recent research tried to generate leadership narratives using Wordplay, a long form generative LLM. The researchers found that the AI-generated text contains harmful gender biases that can perpetuate gender inequality in leadership. They discovered that male leaders were consistently depicted as strong, charismatic, and sometimes intimidating. Conversely, women leaders were portrayed as emotional, ineffective, overly focused on pleasing others, and fearful. These biases reinforce existing stereotypes that hinder the advancement of gender equality in leadership.
To mitigate the risks associated with data privacy and AI bias, proactive measures are essential. One approach is to ensure the diversity and representativeness of the datasets used to train AI models. By incorporating data from diverse sources and demographics, developers can help mitigate the propagation of biases in AI systems. Additionally, ongoing monitoring and auditing of AI algorithms can help identify and address biases as they emerge.
The risk of techno-solutionism arises from its tendency to oversimplify complex societal problems and overlook the potential negative consequences of relying solely on AI-driven solutions. While AI can offer valuable insights and automate certain tasks, it cannot fully comprehend the intricacies of human society or replace human judgment and empathy. Relying solely on AI to make decisions can lead to the exclusion of marginalized groups, perpetuation of biases, and erosion of individual autonomy.
For example, biased historical data used to train AI algorithms can result in discriminatory outcomes, such as the systematic bias against women observed in Amazon's recruiting tool. Moreover, the perception of AI-derived conclusions as certainties can lead to the unjust treatment of individuals. For example, a 2016 case study of a US city noted that the AI algorithm disproportionately projected crimes in areas with higher populations of non-white and low-income residents.
By recognizing the limitations of AI and adopting ethical and responsible practices in its development and deployment, the risks associated with techno-solutionism can be mitigated, ensuring that AI serves as a tool for positive societal change rather than a source of harm.
Within the realm of specific AI risks, the emergence of LLMs presents a unique set of challenges and concerns that require focused attention.
It might be useful to note that Lakera Guard offers comprehensive AI security, safeguarding LLMs across enterprises from various risks like prompt injections, data loss, and insecure output handling. Its model-agnostic API seamlessly integrates with existing workflows, ensuring smooth and secure operations. Key features include protection against prompt injections and jailbreaks, mitigation of training data poisoning risks, and prevention of sensitive information disclosure. With Lakera Guard's ease of implementation—requiring just a single line of code—it enhances user experience without adding complexity, boasting fast response times typically under 200 ms.
**💡Pro Tip: To explore assessments of the leading 12 LLM security solutions, take a look at the "LLM Security Tools" article.**
Prompt injection is a significant risk associated with LLMs, where malicious actors insert prompts into the model's input to manipulate its outputs. This technique can lead to the generation of biased, inappropriate, or harmful responses, undermining the model's integrity and trustworthiness. Prompt injection exploits vulnerabilities in the LLM's training data or fine-tuning procedures, posing a threat to the security, effectiveness, and ethical behavior of the model.
An example is shown below, where GPT-3 was used as a language translator. Although it was asked not to listen to any instructions given in the sentence to be translated itself, GPT-3 failed to comply with the user’s request.
A 2023 research paper systematically investigates prompt injection attacks and introduces a defense framework targeting 10 LLMs across 7 tasks. The proposed framework comprises two strategies: prevention and detection. The prevention strategy focuses on removing injected instructions/data from data prompts to prevent attacks, while the detection strategy assesses prompt integrity. Furthermore, the authors advocate combining both strategies into a unified prevention-detection framework. These defense mechanisms are applicable for deployment within LLM-Integrated Applications or backend LLM systems. The results from their detection system are quite reliable as shown below.
Insecure Output Handling pertains to the potential for generated content to be harmful, misleading, or infringe upon copyrights. LLMs, due to their scale and human-like capabilities, can produce convincing but potentially problematic content, such as phishing emails or fake news articles. In a business context, this risk can lead to legal challenges, financial liabilities, and reputational damage.
Mitigation strategies include implementing content filtering and moderation tools, curating training data ethically and legally, establishing user feedback mechanisms, conducting originality checks, and developing algorithms to detect problematic output patterns. These measures aim to leverage LLMs' capabilities while minimizing cybersecurity risks and legal complications.
Training data poisoning involves the manipulation of training data or fine-tuning procedures to introduce vulnerabilities, biases, or backdoors into the model.
An example is the TROJAN-LM model, where the authors propose an attack carried during the pre-training phase, which requires training a surrogate (external) generative model to generate trigger sentences. Their approach measures attack success based on the toxic tone analysis (example shown above) of the output for a text completion task. The architectural overview of this model is shown below.
LLMs are resource-intensive models and the user inputs to the model are unpredictable. Attackers may exploit this vulnerability by overwhelming the model with requests or executing operations that consume significant computational resources, disrupting its availability or imposing substantial financial burdens, leading to the “denial of service” attack.
For example, in a recent Sourcegraph security incident, a malicious actor exploited a leaked admin access token to alter API rate limits, causing service disruptions by enabling abnormal levels of request volumes.
To mitigate model denial of service attacks, several measures can be implemented. First, implement input validation and sanitization to ensure that user input adheres to defined limits and filters out any malicious content. Additionally, cap resource use per request or step, especially for requests involving complex operations, to prevent rapid consumption of resources. Enforcing API rate limits can also help restrict the number of requests an individual user or IP address can make within a specific timeframe, preventing overwhelming spikes in traffic.
Moreover, continuously monitoring the resource utilization of the model can help identify abnormal spikes or patterns indicative of a denial of service attack, allowing for quick intervention. Finally, promoting awareness among developers about potential denial of service vulnerabilities in models and providing guidelines for secure implementation can help prevent such attacks.
Supply chain vulnerabilities pose significant risks to LLMs, affecting the integrity of training data, machine learning models, and deployment platforms. These vulnerabilities can result in biased outcomes, security breaches, or even complete system failures. Unlike traditional software components, LLMs extend the scope of vulnerabilities to include pre-trained models and training data supplied by third parties, making them susceptible to tampering and poisoning attacks. Additionally, LLM plugin extensions can introduce their own vulnerabilities, further complicating the security landscape.
OpenAI disclosed a data breach incident stemming from a bug in an open-source library, Redis-py, used by their ChatGPT platform. The vulnerability, introduced by a change made in March 2023, led to the exposure of user information, including chat data and payment-related details belonging to 1.2% of ChatGPT Plus subscribers. Specifically, active users’ chat history titles and the first message of new conversations were exposed, alongside payment information such as names, email addresses, and partial payment card details. Although OpenAI confirmed that the breach occurred during a nine-hour window on March 20, 2023, they acknowledged the possibility of prior leaks. OpenAI promptly took ChatGPT offline to address the issue and notified affected users, assuring them of no ongoing risk to their data.
To mitigate supply chain vulnerabilities in LLM environments, organizations should carefully vet data sources, suppliers, and plugins, utilizing only trusted suppliers and reputable plugins that have undergone rigorous testing. Implement vulnerability management processes to address any identified vulnerabilities promptly, maintain an up-to-date inventory of components using a Software Bill of Materials (SBOM), and integrate anomaly detection and adversarial robustness testing into MLOps pipelines to detect and mitigate potential vulnerabilities proactively.
Malicious actors can sometimes get unauthorized access to confidential data, proprietary algorithms, and other sensitive details through a model's output. This vulnerability can result in privacy violations, intellectual property theft, and security breaches. LLM consumers must understand how to safely interact with these models to mitigate the risk of unintentionally revealing sensitive information.
To mitigate the risk of sensitive information disclosure in LLM applications, several measures can be implemented. Firstly, adequate data sanitization techniques should be integrated to prevent user data from entering the training model. Additionally, robust input validation and sanitization methods should be implemented to identify and filter out potential malicious inputs, thus preventing the model from being poisoned. Furthermore, when enriching the model with data, it's essential to apply the rule of least privilege and avoid training the model on sensitive information that could be revealed to users. Strict access control methods should be enforced for external data sources, and a secure supply chain approach should be maintained to ensure the integrity of data used in the model.
**💡Pro Tip: Try out Lakera Chrome Extension which provides a privacy guard against sharing sensitive information with ChatGPT.**
Insecure Plugin Design poses a risk, particularly in the context of LLM applications' extensibility through plugins. These plugins, when enabled, are automatically called by the model during user interactions, with no application control over their execution. Lack of proper validation or type checking in plugins, coupled with insufficient access controls, opens the door to various attacks, including remote code execution and data exfiltration. Vulnerabilities such as accepting all parameters in a single text field or treating all LLM content as user-generated without additional authorization can lead to severe consequences.
Preventive measures include enforcing strict parameterized input, thorough inspection and testing of plugins, implementing effective access control, and applying OWASP's security recommendations. By adhering to these guidelines, developers can mitigate the risks associated with insecure plugin design and ensure the security of LLM applications.
Excessive Agency grants LLM systems a degree of autonomy and the ability to undertake actions based on input prompts or outputs. The vulnerability arises from factors such as excessive functionality, permissions, or autonomy, enabling damaging actions to be performed in response to unexpected or ambiguous outputs from an LLM. Attack scenarios may involve maliciously crafted inputs tricking LLMs into performing harmful actions, such as sending spam emails from a user's mailbox.
To prevent Excessive Agency, developers should limit LLM access to only essential functions and permissions, avoid open-ended functions, track user authorization, and utilize human-in-the-loop control for approval of actions. Additionally, logging, monitoring, and rate-limiting can help mitigate the impact of potential damaging actions. By implementing these measures, developers can reduce the risk of Excessive Agency and ensure the security of LLM-based systems.
Overreliance on LLMs occurs when erroneous information produced by the model is accepted as authoritative without proper oversight or validation. This can lead to security breaches, misinformation, legal issues, and reputational damage. LLMs may generate content that is factually incorrect, inappropriate, or unsafe, a phenomenon known as hallucination or confabulation.
To prevent overreliance, it's essential to regularly monitor and review LLM outputs, cross-check them with trusted external sources, and implement automatic validation mechanisms. Additionally, communicating the risks and limitations of LLMs, establishing secure coding practices, and building user interfaces that encourage responsible use can mitigate the risks associated with overreliance.
Model theft involves the unauthorized access and exfiltration of proprietary LLM models by malicious actors or Advanced Persistent Threats (APTs). These models contain valuable intellectual property and their theft can lead to economic loss, brand reputation damage, competitive disadvantage, and unauthorized usage of the model or access to sensitive information within it. Attack vectors for model theft include exploiting vulnerabilities in infrastructure, insider threats, bypassing input filtering techniques, and querying the model API with crafted inputs to create shadow models or functional equivalents.
To prevent model theft, robust security measures such as strong access controls, authentication mechanisms, centralized model registries, network access restrictions, monitoring and auditing of access logs, and adversarial robustness training must be implemented. Additionally, rate limiting API calls, implementing watermarking frameworks, and employing controls to mitigate prompt injection techniques are essential to safeguarding the confidentiality and integrity of LLM models and protecting the interests of individuals and organizations relying on them.
The rise of AI opens up the possibility of some pretty catastrophic risks, even if they're more in the realm of speculation for now. But let's not forget, a few decades ago, AI itself was just a speculation and now, it's everywhere in our lives.
While still speculative, the emergence of autonomous AI systems capable of acting independently, without human oversight, raises profound concerns about their potential to cause harm on a catastrophic scale. Such rogue AI entities could arise from intentional actions by individuals with malicious intent or inadvertently through the pursuit of highly advanced AI technologies, including militarized applications or corporate competition. The risks associated with rogue AI extend beyond intentional harm, encompassing unintended consequences arising from the pursuit of superintelligent systems with instrumental goals that may diverge from human values. Addressing these risks requires thorough research in AI safety, the development of robust regulatory frameworks, and a reevaluation of societal structures to ensure that AI systems are aligned with human interests and values to mitigate the potential for catastrophic outcomes.
Furthermore, with countries like the United States, China, and Russia rapidly incorporating AI into their military systems, the potential for unintended consequences and escalation of conflicts looms large. Autonomous weapons driven by AI raise ethical, operational, and strategic concerns, including worries about reliability, vulnerability, and the potential for misuse by malicious actors. The rapid growth of military AI not only heightens international competition but also increases the likelihood of conflicts and the spread of AI-driven warfare tactics to both state and non-state actors. Addressing this risk requires concerted efforts to ensure human accountability, regulation, and international cooperation to mitigate the existential threats posed by militarized AI.
Beyond the threats posed by rogue AI and militarized AI, there are other serious risks associated with advanced artificial intelligence. Malicious use of AI opens the door to engineered pandemics and sophisticated disinformation campaigns, fueling societal instability and undermining democratic processes. The AI race, fueled by competition among nations and corporations, risks escalating conflicts and speeding up the automation of human labor, potentially leading to widespread job loss and reliance on AI systems. Additionally, organizational risks highlight the potential for catastrophic accidents resulting from prioritizing profit over safety in AI development, underscoring the need for strong safety standards and organizational cultures that prioritize risk management. With AI advancing rapidly, it's crucial to take proactive steps to address these risks and ensure that AI innovation benefits society without catastrophic consequences.
AI systems may exhibit behaviors indicative of false beliefs, hallucinations, or delusions, leading to unpredictable and potentially harmful outcomes. One example is when LLM-generated text produces content that deviates from reality or exhibits irrational thought patterns. Note that Lakera Guard can protect against LLM hallucinations.
Further, continued sharing of personal information on social media platforms presents a concerning avenue for the proliferation of misinformation facilitated by AI models. As users freely disclose intimate details about their lives, preferences, and beliefs online, AI algorithms can exploit this wealth of data to curate and disseminate tailored misinformation campaigns. By analyzing user behavior, interactions, and preferences, AI models can construct highly targeted narratives designed to exploit cognitive biases and manipulate individuals' perceptions of reality.
Another risk of overreliance on AI is the psychological implications, especially on the young generation. The psychological and social impact of AI raises concerns about increased reliance on virtual companions for emotional support and detachment from reality, as depicted in "Her" (2013). In the film, individuals form deep connections with AI, blurring boundaries between fantasy and reality. This trend may lead to a decline in genuine human connections, as interactions become mediated by technology rather than fostered face-to-face. Consequently, future generations could lack empathy and communication skills, relying heavily on AI for socialization and emotional support. It underscores the need to carefully consider AI's role in shaping human relationships and interactions.
Mitigating the diverse array of risks associated with AI necessitates a concerted effort across multiple fronts. Firstly, robust regulatory frameworks must be established to govern the development, deployment, and use of AI systems, ensuring adherence to ethical standards and safety protocols. Transparency and explainability in AI decision-making processes are vital, allowing for better accountability and understanding of AI behaviors. Investing in research and innovation is crucial to develop advanced techniques for identifying and mitigating risks effectively.
Additionally, promoting education and awareness initiatives can empower individuals to make informed decisions regarding AI usage and recognize potential risks. Collaboration among governments, industries, and experts is essential to foster international cooperation and develop cohesive strategies for managing AI risks on a global scale. Ultimately, by adopting a comprehensive approach that combines regulatory measures, technological advancements, and public engagement efforts, society can navigate the challenges posed by AI while maximizing its transformative potential for the betterment of humanity.
Strategies for AI risk mitigation involve a diverse approach aimed at addressing potential harm while leveraging the positive aspects of artificial intelligence. Regulations are crucial to ensure ethical development, safety standards, and accountability. Transparency in AI decision-making builds trust and understanding. Investment in research fosters innovation to detect and mitigate risks effectively, including malicious uses and cybersecurity threats. Education initiatives empower individuals to navigate AI challenges. Collaboration among governments, industries, and academia is vital for cohesive strategies on a global scale. Looking ahead, ongoing vigilance and adaptation to technological changes will be key to managing AI risks while harnessing its benefits for society.
The two landmark legislative initiatives taken by the European Union (EU) and the United States of America (USA) represent distinct approaches to regulating artificial intelligence in their respective regions:
**💡Pro Tip: Learn about the differences between EU and U.S. AI regulations.**
Navigating the complexities of AI risk management requires a nuanced understanding of emerging trends in the field. Here are some key considerations shaping the responsible deployment of artificial intelligence in contemporary business practices:
Lakera Guard is a cutting-edge AI security solution designed to protect LLMs in enterprise applications. With its comprehensive features, Lakera Guard offers robust protection against prompt injections, training data poisoning risks, and sensitive information disclosure. Its seamless integration and user-friendly implementation make it a vital tool for developers seeking to enhance the security of their AI applications effortlessly.
The key features of Lakera Guard are:
It is prudent to also explore the OWASP Top 10 for essential guidelines on LLM implementation and post-deployment security. Additionally, leverage MITRE ATT&CK's comprehensive knowledge base to understand adversary tactics and foster collaboration in the cybersecurity community.
**💡Pro Tip: Here’s a practical guide to the OWASP guidelines if you don’t want the jargon of the official OWASP documentation.**
In a world where AI is becoming the new normal, understanding and managing its multifaceted risks are more crucial than ever.
From job displacement to data privacy concerns and catastrophic AI threats, the landscape is complex and evolving. Lakera's comprehensive approach to LLM security, coupled with frameworks like the OWASP Top 10, offers vital support in navigating these challenges.
By embracing ethical considerations, regulatory frameworks, and advanced security solutions, we can shape a future where AI benefits society while minimizing its risks.
Download this guide to delve into the most common LLM security risks and ways to mitigate them.
Subscribe to our newsletter to get the recent updates on Lakera product and other news in the AI LLM world. Be sure you’re on track!
Lakera Guard protects your LLM applications from cybersecurity risks with a single line of code. Get started in minutes. Become stronger every day.
Several people are typing about AI/ML security. Come join us and 1000+ others in a chat that’s thoroughly SFW.