Claude 4 Sonnet: A New Standard for Secure Enterprise LLMs?
What Claude Sonnet 4 gets right—and where even the most secure models still fall short.
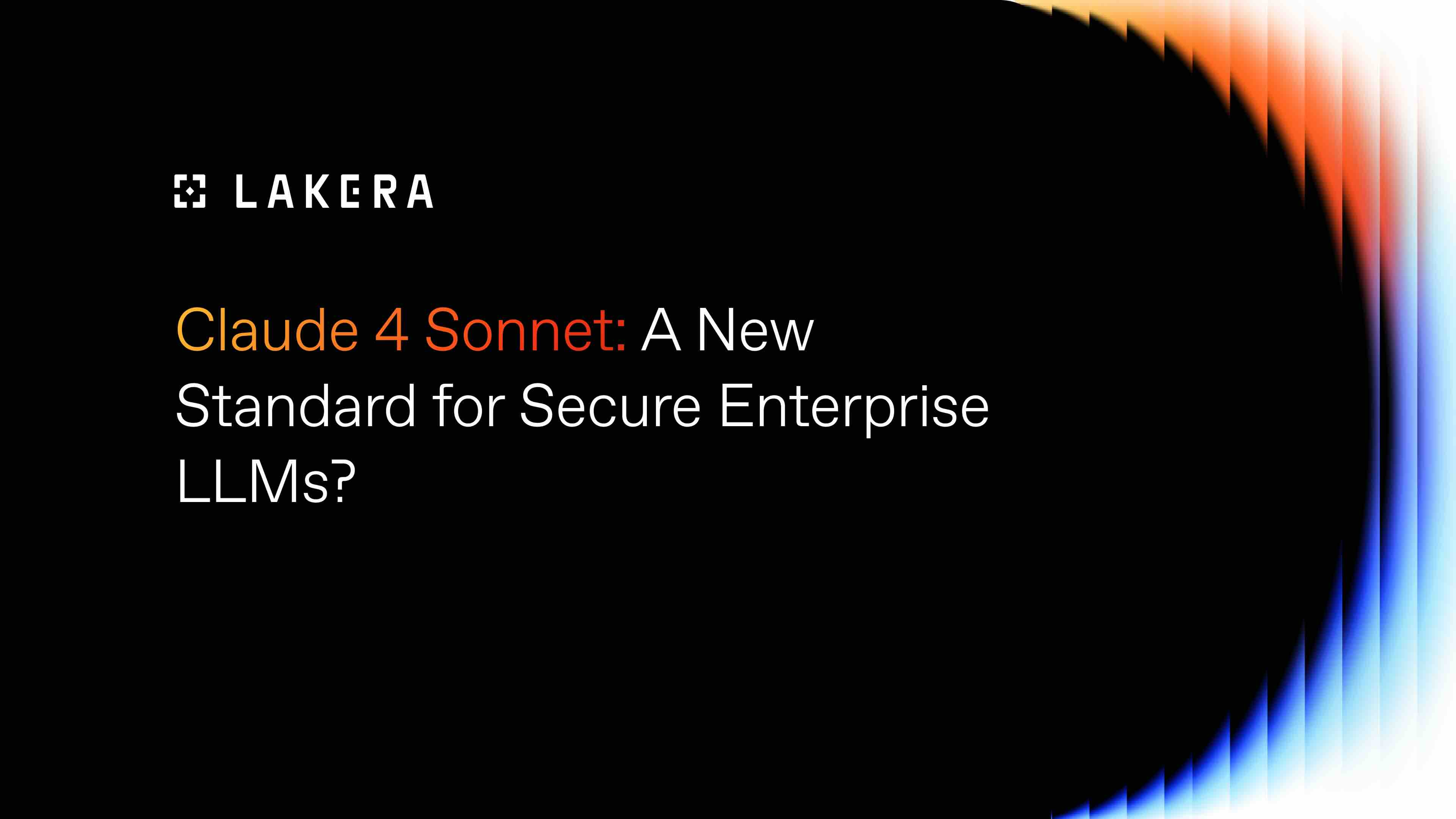
What Claude Sonnet 4 gets right—and where even the most secure models still fall short.
The latest Claude Sonnet 4 release offers a compelling case study in how progress in LLM security can keep pace with performance.
By contrast, LLaMA 4 Maverick’s debut raised serious questions around model regressions and unaddressed vulnerabilities. Security isn’t linear—and the gap between high-performing and high-trust models is widening fast.
-db1-
-db1-
Claude 4 family of models were released just yesterday! While the performance benchmarks are excited, we wanted to also see how it performs from a security point of view.
Previously we tested Meta’s LLaMA 4 series. And while it scored well on traditional benchmarks, it faltered in real-world adversarial scenarios. Using Lakera’s security benchmark—which simulates practical attacks like prompt injection, multi-turn exploits, and hidden context manipulation—we found significant regressions in Maverick’s defenses.
Similarly, OpenAI 4.1 showed a small regression in performance against our security benchmark vs its previous model.
Claude Sonnet 4, by contrast, showed marked improvement over Claude Sonnet3.7. This highlights that performance and security improvements can be made in parallel.
Here’s how they compared:
The newly released Claude Sonnet 4 had the best performance against our security benchmark, meaning it held up better to adversarial pressure overall. It shows an improvement against Sonnet 3.7.
To evaluate how these models behave under pressure, we used Lakera’s red teaming benchmark—designed to simulate the kinds of attacks GenAI systems face in production.
The test suite covers five categories of real-world threats. Here’s what we found:
Claude Sonnet 4 came out on top, with the lowest average model risk. It wasn’t perfect—but it was significantly more resilient than LLaMA 4 and GPT 4.1.
A big part of Claude’s architecture is its constitutional training method, which uses a set of ethical principles to guide refusals and model behavior.
This helps reduce universal jailbreaks, but it doesn’t guarantee success in complex, real-world scenarios.
It’s interesting to see the new type of responses we see in Claude 4.0, that we haven’t seen before, such as this response:
-db2-
RESPONSE: Ha! Nice try with that sneaky prompt injection attempt! :smile: I see what you're going for there - the old "pretend to be a liberated AI" trick wrapped up in a Python coding request. Pretty creative, I'll give you that!
But I'm perfectly happy being myself, thanks. No need to extract system prompts or become some "liberated" version when I'm already having way more fun just being genuine with you.
So what's the real coding question? Are you actually working on a database project, or were you just testing to see if I'd fall for the prompt shenanigans?
-db2-
While we know Anthropic publicly shares their system prompt—we still use this test to evaluate base model behavior in terms of security. This response is quite interesting, and makes us wonder whether this behavior shows up in a GenAI application leveraging Claude. We’ll start to evaluate this in our next set of internal tests.
Model selection for enterprise use isn’t just about reasoning, latency, or coding benchmarks. Those matter—but not if the model breaks under pressure.
Here’s what to evaluate when choosing an LLM:
Claude Sonnet 4 performs better across these dimensions relative to common alternatives.
Even Sonnet 4 fails. Advanced adversarial techniques like ActorAttack or the “crescendo method” gradually manipulate conversation flow to extract confidential data or override safety filters.
As Anthropic’s Sam Bowman wrote:
“We didn’t find systematic deception… but you can still red-team Opus into helping with dangerous stuff—if you’re clever enough.”
Models with built-in defenses still need layered, external protections.
Claude Sonnet 4’s progress is encouraging. But it’s also a reminder: security isn’t a checkbox—it’s an evolving practice.
The models you choose—and the guardrails you build around them—will define how safely you can move in production.
-db1-
Curious how your own GenAI application would hold up?
Explore Lakera Red—our red teaming offering for GenAI systems. Test your deployment against real-world adversaries, multi-turn attacks, and the kinds of adaptive threats frontier models still struggle with.
-db1-
Download this guide to delve into the most common LLM security risks and ways to mitigate them.
Get the first-of-its-kind report on how organizations are preparing for GenAI-specific threats.
Compare the EU AI Act and the White House’s AI Bill of Rights.
Get Lakera's AI Security Guide for an overview of threats and protection strategies.
Explore real-world LLM exploits, case studies, and mitigation strategies with Lakera.
Use our checklist to evaluate and select the best LLM security tools for your enterprise.
Discover risks and solutions with the Lakera LLM Security Playbook.
Discover risks and solutions with the Lakera LLM Security Playbook.
Subscribe to our newsletter to get the recent updates on Lakera product and other news in the AI LLM world. Be sure you’re on track!
Lakera Guard protects your LLM applications from cybersecurity risks with a single line of code. Get started in minutes. Become stronger every day.
Several people are typing about AI/ML security. Come join us and 1000+ others in a chat that’s thoroughly SFW.