LLM Hallucinations
In the context of Large Language Models (LLMs), hallucination refers to the phenomenon where the model produces text that is incorrect, nonsensical, or unfounded. It's not a result of the model accessing real or factual information, but rather an extrapolation or generation based on its training and the given prompt. These outputs, while sounding plausible, might not be rooted in any factual or real-world information.
LLM Hallucinations in practice
- Statistical Patterns: Similar to the Markov model example, LLMs rely on statistical patterns ingrained from their training data. This means the generated text is based on the most correlated or likely sequence from the prompt, even if it leads to incorrect or made-up content.
- Limited Contextual Understanding: LLMs operate by transforming the given prompt and their vast training data into an abstract representation. In this process, some nuances or specifics might be lost, leading the model to generate text that may not always align with real-world facts.
To counter hallucinations, techniques such as controlled generation can be used. By providing a more detailed and constrained prompt, the model is given less freedom to diverge into hallucinatory content. Another approach involves human oversight to review, correct, or guide the model's output.
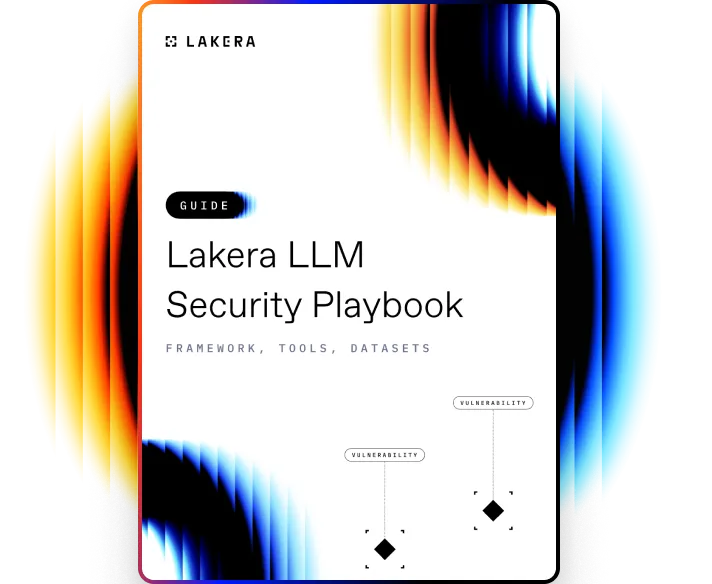
Download this guide to delve into the most common LLM security risks and ways to mitigate them.

untouchable mode.
Lakera Guard protects your LLM applications from cybersecurity risks with a single line of code. Get started in minutes. Become stronger every day.
Several people are typing about AI/ML security. 
Come join us and 1000+ others in a chat that’s thoroughly SFW.