Federated Learning
Federated Learning is a machine learning approach that allows a model to be trained across multiple devices or servers holding local data samples without exchanging their actual data. This decentralized training process can improve privacy, security, and use of device resources.
How Federated Learning works
In Federated Learning, the training process begins with a global model being sent to all participating devices or nodes in the network. Each node computes a model update based on its local data. These updates, not the raw data, are then sent back to a central server where they are aggregated to update the global model. This iterative process of sending the global model to each node for local updates and then aggregating those updates, is repeated until the model is sufficiently trained.
Federated Learning is particularly useful for privacy-sensitive applications since local data never needs to leave the original device. It can also help reduce the amount of data being sent over the network, as only model updates need to be transmitted, not the complete datasets. This makes Federated Learning an efficient way to build machine learning models where data privacy and security is crucial or where network bandwidth may be limited.
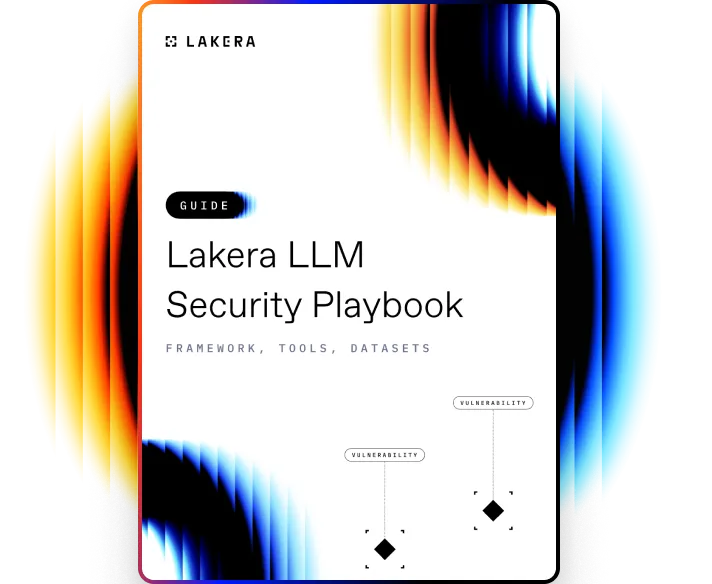
Download this guide to delve into the most common LLM security risks and ways to mitigate them.

untouchable mode.
Lakera Guard protects your LLM applications from cybersecurity risks with a single line of code. Get started in minutes. Become stronger every day.
Several people are typing about AI/ML security. 
Come join us and 1000+ others in a chat that’s thoroughly SFW.